10 Connecting conceptualization and measurement
Chapter Outline
- Measurement Modeling (17-minute read)
- Critiquing Measurement Models (23-minute read)
- Positionality & Reflexivity (19-minute read)
- Assumptions of the Postpositivist Paradigm (30-minute read)
Content warning: TBD.
10.1 Measurement Modeling
Learning Objectives
Learners will be able to…
- Define measurement modeling and how it relates to conceptual and operational definitions
- Describe how operational definitions are impacted by power.
- Apply validity and reliability to measurement models
With your variables operationalized, it’s time to take a step back and look at the assumptions underlying operational definitions and conceptual definitions. In addition to the validity and reliability concerns we reviewed in the previous chapter, how we measure things is both shaped by power arrangements inside our society, and more insidiously, by establishing what is scientifically true, measures have their own power to influence the world. Just like reification in the conceptual world, how we operationally define concepts can reinforce or fight against oppressive forces.
Separating concepts from their measurement in empirical studies
Measurement in social science often involve unobservable theoretical constructs, such as socioeconomic status, teacher effectiveness, and risk of recidivism. As we discussed in Chapter 8, such constructs cannot be measured directly and must instead be inferred from measurements of observable properties (and other unobservable theoretical constructs) thought to be related to them—i.e., operationalized via a measurement model. This process, which necessarily involves making assumptions, introduces the potential for mismatches between the theoretical understanding of the construct purported to be measured and its operationalization.
Many of the harms discussed in the literature on fairness in computational systems are direct results of such mismatches. Some of these harms could have been anticipated and, in some cases, mitigated if viewed through the lens of measurement modeling. To do this, we contribute fairness oriented conceptualizations of construct validity that provide a set of tools for making explicit and testing assumptions about constructs and their operationalizations.
In essence, we want to make sure that the measures selected for a research project match with the conceptualization for that research project. Novice researchers and practitioners are often inclined to conflate constructs and their operationalization definitions—i.e., to collapse the distinctions between someone’s anxiety and their score on the GAD-7 Anxiety inventory. But collapsing these distinctions, either colloquially or epistemically, makes it difficult to anticipate, let alone mitigate, any possible mismatches. When reading a research study, you should be able to see how the researcher’s conceptualization informed what indicators and measurements were used. Collapsing the distinction between conceptual definitions and operational definitions is when fairness-related harms are most often introduced into the scientific process.
Making assumptions when measuring
Measurement modeling plays a central role in the quantitative social sciences, where many theories involve unobservable theoretical constructs—i.e., abstractions that describe phenomena of theoretical interest. For example, researchers in psychology and education have long been interested in studying intelligence, while political scientists and sociologists are often concerned with political ideology and socioeconomic status, respectively. Although these constructs do not manifest themselves directly in the world, and therefore cannot be measured directly, they are fundamental to society and thought to be related to a wide range of observable properties
A measurement model is a statistical model that links unobservable theoretical constructs, operationalized as latent variables, and observable properties—i.e., data about the world [30]. In this section, we give a brief overview of the measurement modeling process, starting with two comparatively simple examples—measuring height and measuring socioeconomic status—before moving on to three well-known examples from the literature on fairness in computational systems. In emphasizing computational systems, we wish to call attention to how operational definitions are often imposed on clients and community members without their consent. Our goal in this section is not to provide comprehensive mathematical details for each of our five examples, but instead to introduce key terminology and, more importantly, to highlight that the measurement modeling process necessarily involves making assumptions that must be made explicit and tested before the resulting measurements are used.
Assumptions of measuring height
We start by formalizing the process of measuring the height of a person—a property that is typically thought of as being observable and therefore easy to measure directly. There are many standard tools for measuring height, including rulers, tape measures, and height rods. Indeed, measurements of observable properties like height are sometimes called representational measurements because they are derived by “representing physical objects [such as people and rulers] and their relationships by numbers” [25]. Although the height of a person is not an unobservable theoretical construct, for the purpose of exposition, we refer to the abstraction of height as a construct H and then operationalize H as a latent variable h.
Despite the conceptual simplicity of height—usually understood to be the length from the bottom of a person’s feet to the top of their head when standing erect—measuring it involves making several assumptions, all of which are more or less appropriate in different contexts and can even affect different people in different ways. For example, should a person’s hair contribute to their height? What about their shoes? Neither are typically viewed as being an intrinsic part of a person’s height, yet both contribute to a person’s effective height, which may matter more in ergonomic contexts. Similarly, if a person uses a wheelchair, then their standing height may be less relevant than their sitting height. These assumptions must be made explicit and tested before using any measurements that depend upon them.
In practice, it is not possible to obtain error-free measurements of a person’s height, even when using standard tools. For example, when using a ruler, the angle of the ruler, the granularity of the marks, and human error can all result in erroneous measurements. However, if we take many measurements of a person’s height, then provided that the ruler is not statistically biased, the average will converge to the person’s “true” height h. If we were to measure them infinite times, we would be able to measure their exact height perfectly. with our probability of doing so increasing the more times we measure.
In our measurement model, we say that the person’s true height—the latent variable h—influences the measurements every time we observe it. We refer to models that formalize the relationships between measurements and their errors as measurement error models. In many contexts, it is reasonable to assume that the errors associated will not impact the consistency or accuracy of a measure as long as the error is normally distributed, statistically unbiased, and possessing small variance. However, in some contexts, the measurement error may not behave like researcher expect and may even be correlated with demographic factors, such as race or gender.
As an example, suppose that our measurements come not from a ruler but instead from self-reports on dating websites. It might initially seem reasonable to assume that the corresponding errors are well-behaved in this context. However, Toma et al. [54] found that although men and women both over-report their height on dating websites, men are more likely to over-report and to over-report by a larger amount. Toma et al. suggest this is strategic, likely representing intentional deception. However, regardless of the cause, these errors are not well-behaved and are correlated with gender. Assuming that they are well-behaved will yield inaccurate measurements.
Measuring socioeconomic status
We now consider the process of measuring a person’s socioeconomic status (SES). From a theoretical perspective, a person’s SES is understood as encompassing their social and economic position in relation to others. Unlike a person’s height, their SES is unobservable, so it cannot be measured directly and must instead be inferred from measurements of observable properties (and other unobservable theoretical constructs) thought to be related to it, such as income, wealth, education, and occupation. Measurements of phenomena like SES are sometimes called pragmatic measurements because they are designed to capture particular aspects of a phenomenon for particular purposes [25].
We refer to the abstraction of SES as a construct S and then operationalize S as a latent variable s. The simplest way to measure a person’s SES is to use an observable property—like their income—as an indicator for it. Letting the construct I represent the abstraction of income and operationalizing I as a latent variable i, this means specifying a both measurement model that links s and i and a measurement error model. For example, if we assume that s and i are linked via the identity function—i.e., that s = i—and we assume that it is possible to obtain error-free measurements of a person’s income—i.e., that ˆi = i—then s = ˆi. Like the previous example, this example highlights that the measurement modeling process necessarily involves making assumptions. Indeed, there are many other measurement models that use income as a proxy for SES but make different assumptions about the specific relationship between them.
Similarly, there are many other measurement error models that make different assumptions about the errors that occur when measuring a person’s income. For example, if we measure a person’s monthly income by totaling the wages deposited into their account over a single one-month period, then we must use a measurement error model that accounts for the possibility that the timing of the one-month period and the timings of their wage deposits may not be aligned. Using a measurement error model that does not account for this possibility—e.g., using ˆi = i—will yield inaccurate measurements.
Human Rights Watch reported exactly this scenario in the context of the Universal Credit benefits system in the U.K. [55]: The system measured a claimant’s monthly income using a one-month rolling period that began immediately after they submitted their claim without accounting for the possibility described above. This meant that the system “might detect that an individual received a £1000 paycheck on March 30 and another £1000 on April 29, but not that each £1000 salary is a monthly wage [leading it] to compute the individual’s benefit in May based on the incorrect assumption that their combined earnings for March and April (i.e., £2000) are their monthly wage,” denying them much-needed resources. Moving beyond income as a proxy for SES, there are arbitrarily many ways to operationalize SES via a measurement model, incorporating both measurements of observable properties, such as wealth, education, and occupation, as well as measurements of other unobservable theoretical constructs, such as cultural capital.
Measuring teacher effectiveness
At the end of every semester, students in just about every university classroom in the United States complete similar student evaluations of teaching (SETs). Since every student is likely familiar with these, we can recognize many of the concepts we discussed in the previous sections. There are number of rating scale questions that ask you to rate the professor, class, and teaching effectiveness on a scale of 1-5. Scores are averaged across students and used to determine the quality of teaching delivered by the faculty member. SETs scores are often a principle component of how faculty are reappointed to teaching positions. Would it surprise you to learn that student evaluations of teaching are of questionable quality? If your instructors are assessed with a biased or incomplete measure, how might that impact your education?
Most often, student scores are averaged across questions and reported as a final average. This average is used as one factor, often the most important factor, in a faculty member’s reappointment to teaching roles. We learned in the previous chapter that rating scales are ordinal, not interval or ratio, and the data are categories not numbers. Although rating scales use a familiar 1-5 scale, the numbers 1, 2, 3, 4, & 5 are really just helpful labels for categories like “excellent” or “strongly agree.” If we relabeled these categories as letters (A-E) rather than as numbers (1-5), how would you average them?
Averaging ordinal data is methodologically dubious, as the numbers are merely a useful convention. As you will learn in Chapter 14, taking the median value is what makes the most sense with ordinal data. Median values are also less sensitive to outliers. So, a single student who has strong negative or positive feelings towards the professor could bias the class’s SETs scores higher or lower than what the “average” student in the class would say, particularly for classes with few students or in which fewer students completed evaluations of their teachers.
We care about teaching quality because more effective teachers will produce more knowledgeable and capable students. However, student evaluations of teaching are not particularly good indicators of teaching quality and are not associated with the independently measured learning gains of students (i.e., test scores, final grades) (Uttl et al., 2017).[1] This speaks to the lack of criterion validity. Higher teaching quality should be associated with better learning outcomes for students, but across multiple studies stretching back years, there is no association that cannot be better explained by other factors. To be fair, there are scholars who find that SETs are valid and reliable. For a thorough defense of SETs as well as a historical summary of the literature see Benton & Cashin (2012).[2]
Even though student evaluations of teaching often contain dozens of questions, researchers often find that the questions are so highly interrelated that one concept (or factor, as it is called in a factor analysis) explains a large portion of the variance in teachers’ scores on student evaluations (Clayson, 2018).[3] Personally, I believe based on completing SETs myself that factor is probably best conceptualized as student satisfaction, which is obviously worthwhile to measure, but is conceptually quite different from teaching effectiveness or whether a course achieved its intended outcomes. The lack of a clear operational and conceptual definition for the variable or variables being measured in student evaluations of teaching also speaks to a lack of content validity. Researchers check content validity by comparing the measurement method with the conceptual definition, but without a clear conceptual definition of the concept measured by student evaluations of teaching, it’s not clear how we can know our measure is valid. Indeed, the lack of clarity around what is being measured in teaching evaluations impairs students’ ability to provide reliable and valid evaluations. So, while many researchers argue that the class average SETs scores are reliable in that they are consistent over time and across classes, it is unclear what exactly is being measured even if it is consistent (Clayson, 2018).[4]
As a faculty member, there are a number of things I can do to influence my evaluations and disrupt validity and reliability. Since SETs scores are associated with the grades students perceive they will receive (e.g., Boring et al., 2016),[5] guaranteeing everyone a final grade of A in my class will likely increase my SETs scores and my chances at tenure and promotion. I could time an email reminder to complete SETs with releasing high grades for a major assignment to boost my evaluation scores. On the other hand, student evaluations might be coincidentally timed with poor grades or difficult assignments that will bias student evaluations downward. Students may also infer I am manipulating them and give me lower SET scores as a result. To maximize my SET scores and chances and promotion, I also need to select which courses I teach carefully. Classes that are more quantitatively oriented generally receive lower ratings than more qualitative and humanities-driven classes, which makes my decision to teach social work research a poor strategy (Uttl & Smibert, 2017).[6] The only manipulative strategy I will admit to using is bringing food (usually cookies or donuts) to class during the period in which students are completing evaluations. Measurement is impacted by context.
As a white cis-gender male educator, I am adversely impacted by SETs because of their sketchy validity, reliability, and methodology. The other flaws with student evaluations actually help me while disadvantaging teachers from oppressed groups. Heffernan (2021)[7] provides a comprehensive overview of the sexism, racism, ableism, and prejudice baked into student evaluations:
“In all studies relating to gender, the analyses indicate that the highest scores are awarded in subjects filled with young, white, male students being taught by white English first language speaking, able-bodied, male academics who are neither too young nor too old (approx. 35–50 years of age), and who the students believe are heterosexual. Most deviations from this scenario in terms of student and academic demographics equates to lower SET scores. These studies thus highlight that white, able-bodied, heterosexual, men of a certain age are not only the least affected, they benefit from the practice. When every demographic group who does not fit this image is significantly disadvantaged by SETs, these processes serve to further enhance the position of the already privileged” (p. 5).
The staggering consistency of studies examining prejudice in SETs has led to some rather superficial reforms like reminding students to not submit racist or sexist responses in the written instructions given before SETs. Yet, even though we know that SETs are systematically biased against women, people of color, and people with disabilities, the overwhelming majority of universities in the United States continue to use them to evaluate faculty for promotion or reappointment. From a critical perspective, it is worth considering why university administrators continue to use such a biased and flawed instrument. SETs produce data that make it easy to compare faculty to one another and track faculty members over time. Furthermore, they offer students a direct opportunity to voice their concerns and highlight what went well.
As the people with the greatest knowledge about what happened in the classroom as whether it met their expectations, providing students with open-ended questions is the most productive part of SETs. Personally, I have found focus groups written, facilitated, and analyzed by student researchers to be more insightful than SETs. MSW student activists and leaders may look for ways to evaluate faculty that are more methodologically sound and less systematically biased, creating institutional change by replacing or augmenting traditional SETs in their department. There is very rarely student input on the criteria and methodology for teaching evaluations, yet students are the most impacted by helpful or harmful teaching practices.
Students should fight for better assessment in the classroom because well-designed assessments provide documentation to support more effective teaching practices and discourage unhelpful or discriminatory practices. Flawed assessments like SETs, can lead to a lack of information about problems with courses, instructors, or other aspects of the program. Think critically about what data your program uses to gauge its effectiveness. How might you introduce areas of student concern into how your program evaluates itself? Are there issues with food or housing insecurity, mentorship of nontraditional and first generation students, or other issues that faculty should consider when they evaluate their program? Finally, as you transition into practice, think about how your agency measures its impact and how it privileges or excludes client and community voices in the assessment process.
Let’s consider an example from social work practice. Let’s say you work for a mental health organization that serves youth impacted by community violence. How should you measure the impact of your services on your clients and their community? Schools may be interested in reducing truancy, self-injury, or other behavioral concerns. However, by centering delinquent behaviors in how we measure our impact, we may be inattentive to the role of trauma, family dynamics, and other cognitive and social processes beyond “delinquent behavior.” Indeed, we may bias our interventions by focusing on things that are not as important to clients’ needs. Social workers want to make sure their programs are improving over time, and we rely on our measures to indicate what to change and what to keep. If our measures present a partial or flawed view, we lose our ability to establish and act on scientific truths.
SETs are important to me a a faculty member. For students, you may be interested in similar arguments against the standard grading scale (A-F), and why grades (numerical, letter, etc.) do not do a good job of measuring learning. Think critically about the role that grades play in your life as a student, your self-concept, and your relationships with teachers. Your test and grade anxiety is due in part to how your learning is measured. Those measurements end up becoming an official record of your scholarship and allow employers or funders to compare you to other scholars. The stakes for measurement are the same for participants in your research study.
After you graduate, social work licensing examinations govern entry-to-practice regulations for most clinicians. I wrote a commentary article addressing potential racial bias in social work licensing exams. If you are interested in an example of missing or flawed measures that relates to systems your social work practice is governed by (rather than SETs which govern our practice in higher education) check it out!
Key Takeaways
- Mismatches between conceptualization and measurement are often places in which bias and systemic injustice enter the research process.
- Measurement modeling is a way of foregrounding researcher’s assumptions in how they connect their conceptual definitions and operational definitions.
Exercises
- Evaluate the measurement model in your research study.
- Provide a measurement error model for your conceptual framework.
10.2 Critically evaluating the assumptions of measurement models
Learning Objectives
Learners will be able to…
- Apply
- Second
Diving into measurement modeling further, we will consider another well-known example from the literature on fairness in computational systems: the risk assessment models used in the U.S. justice system to measure a defendant’s risk of recidivism. There are many such models, but we focus here on Northpointe’s Correctional Offender Management Profiling for Alternative Sanctions (COMPAS), which was the subject of an investigation by Angwin et al. [4] and many academic papers [e.g., 9, 14, 34].
COMPAS draws on several criminological theories to operationalize a defendant’s risk of recidivism using measurements of a variety of observable properties (and other unobservable theoretical constructs) derived from official records and interviews. These properties and measurements span four different dimensions: prior criminal history, criminal associates, drug involvement, and early indicators of juvenile delinquency problems [19]. The measurements are combined in a regression model, which outputs a score that is converted to a number between one and ten with ten being the highest risk. Although the full mathematical details of COMPAS are not readily available, the COMPAS documentation mentions numerous assumptions, the most important of which is that recidivism is defined as “a new misdemeanor or felony arrest within two years.” We discuss the implications of this assumption after we introduce our second example.
Finally, we turn to a different type of risk assessment model, used in the U.S. healthcare system to identify the patients that will benefit the most from enrollment in high-risk care management programs— i.e., programs that provide access to additional resources for patients with complex health issues. As explained by Obermeyer et al., these models assume that “those with the greatest care needs will benefit the most from the programs” [43]. Furthermore, many of them operationalize greatest care needs as greatest care costs. This assumption—i.e., that care costs are a reasonable proxy for care needs—transforms the difficult task of measuring the extent to which a patient will benefit from a program (an unobservable theoretical construct) into the simpler task of predicting their future care costs based on their past care costs (an observable property). However, this assumption masks an important confounding factor: patients with comparable past care needs but different access to care will likely have different past care costs. As we explain in the next section, even without considering any other details of these models, this assumption can lead to fairness-related harms.
The measurement modeling process necessarily involves making assumptions. However, these assumptions must be made explicit and tested before the resulting measurements are used. Leaving them implicit or untested obscures any possible mismatches between the theoretical understanding of the construct purported to be measured and its operationalization, in turn obscuring any resulting fairness-related harms. In this section we apply and extend the measurement quality concepts from Chapter 9 to address specifically aspects of fairness and social justice.
Construct Validity
Construct validity is roughly analogous to the concept of statistical unbiasedness [30]. Establishing construct validity means demonstrating, in a variety of ways, that the measurements obtained from measurement model are both meaningful and useful: Does the operationalization capture all relevant aspects of the construct purported to be measured? Do the measurements look plausible? Do they correlate with other measurements of the same construct? Or do they vary in ways that suggest that the operationalization may be inadvertently capturing aspects of other constructs? Are the measurements predictive of measurements of any relevant observable properties (and other unobservable theoretical constructs) thought to be related to the construct, but not incorporated into the operationalization? Do the measurements support known hypotheses about the construct? What are the consequences of using the measurements—including any societal impacts [40, 52]. We emphasize that a key feature, not a bug, of construct validity is that it is not a yes/no box to be checked: construct validity is always a matter of degree, to be supported by critical reasoning [36].
Different disciplines have different conceptualizations of construct validity, each with its own rich history. For example, in some disciplines, construct validity is considered distinct from content validity and criterion validity, while in other disciplines, content validity and criterion validity are grouped under the umbrella of construct validity. Our conceptualization unites traditions from political science, education, and psychology by bringing together the seven different aspects of construct validity that we describe below. We argue that each of these aspects plays a unique and important role in understanding fairness in computational systems.
Face validity
Face validity refers to the extent to which the measurements obtained from a measurement model look plausible— a “sniff test” of sorts. This aspect of construct validity is inherently subjective, so it is often viewed with skepticism if it is not supplemented with other, less subjective evidence. However, face validity is a prerequisite for establishing construct validity: if the measurements obtained from a measurement model aren’t facially valid, then they are unlikely to possess other aspects of construct validity.
It is likely that the models described thus far would yield measurements that are, for the most part, facially valid. For example, measurements obtained by using income as a proxy for SES would most likely possess face validity. SES and income are certainly related and, in general, a person at the high end of the income distribution (e.g., a CEO) will have a different SES than a person at the low end (e.g., a barista). Similarly, given that COMPAS draws on several criminological theories to operationalize a defendant’s risk of recidivism, it is likely that the resulting scores would look plausible. One exception to this pattern is the EVAAS MRM. Some scores may look plausible—after all, students’ test scores are not unrelated to teacher effectiveness—but the dramatic variability that we described above in the context of test–retest reliability is implausible.
Content validity
Content validity refers to the extent to which an operationalization wholly and fully captures the substantive nature of the construct purported to be measured. This aspect of construct validity has three sub-aspects, which we describe below.
The first sub-aspect relates to the construct’s contestedness. If a construct is essentially contested then it has multiple context dependent, and sometimes even conflicting, theoretical understandings. Contestedness makes it inherently hard to assess content validity: if a construct has multiple theoretical understandings, then it is unlikely that a single operationalization can wholly and fully capture its substantive nature in a meaningful fashion. For this reason, some traditions make a single theoretical understanding of the construct purported to be measured a prerequisite for establishing content validity [25, 30]. However, other traditions simply require an articulation of which understanding is being operationalized [53]. We take the perspective that the latter approach is more practical because it is often the case that unobservable theoretical constructs are essentially contested, yet we still wish to measure them.
Of the models described previously, most are intended to measure unobservable theoretical constructs that are (relatively) uncontested. One possible exception is patient benefit, which can be understood in a variety of different ways. However, the understanding that is operationalized in most high-risk care management enrollment models is clearly articulated. As Obermeyer et al. explain, “[the patients] with the greatest care needs will benefit the most” from enrollment in high-risk care management programs [43].
The second sub-aspect of content validity is sometimes known as substantive validity. This sub-aspect moves beyond the theoretical understanding of the construct purported to be measured and focuses on the measurement modeling process—i.e., the assumptions made when moving from abstractions to mathematics. Establishing substantive validity means demonstrating that the operationalization incorporates measurements of those—and only those—observable properties (and other unobservable theoretical constructs, if appropriate) thought to be related to the construct. For example, although a person’s income contributes to their SES, their income is by no means the only contributing factor. Wealth, education, and occupation all affect a person’s SES, as do other unobservable theoretical constructs, such as cultural capital. For instance, an artist with significant wealth but a low income should have a higher SES than would be suggested by their income alone.
As another example, COMPAS defines recidivism as “a new misdemeanor or felony arrest within two years.” By assuming that arrests are a reasonable proxy for crimes committed, COMPAS fails to account for false arrests or crimes that do not result in arrests [50]. Indeed, no computational system can ever wholly and fully capture the substantive nature of crime by using arrest data as a proxy. Similarly, high-risk care management enrollment models assume that care costs are a reasonable proxy for care needs. However, a patient’s care needs reflect their underlying health status, while their care costs reflect both their access to care and their health status.
Finally, establishing structural validity, the third sub-aspect of content validity, means demonstrating that the operationalization captures the structure of the relationships between the incorporated observable properties (and other unobservable theoretical constructs, if appropriate) and the construct purported to be measured, as well as the interrelationships between them [36, 40].
In addition to assuming that teacher effectiveness is wholly and fully captured by students’ test scores—a clear threat to substantive validity [2]—the EVAAS MRM assumes that a student’s test score for subject j in grade k in year l is approximately equal to the sum of the state or district’s estimated mean score for subject j in grade k in year l and the student’s current and previous teachers’ effects (weighted by the fraction of the student’s instructional time attributed to each teacher). However, this assumption ignores the fact that, for many students, the relationship may be more complex.
Convergent validity
Convergent validity refers to the extent to which the measurements obtained from a measurement model correlate with other measurements of the same construct, obtained from measurement models for which construct validity has already been established. This aspect of construct validity is typically assessed using quantitative methods, though doing so can reveal qualitative differences between different operationalizations.
We note that assessing convergent validity raises an inherent challenge: “If a new measure of some construct differs from an established measure, it is generally viewed with skepticism. If a new measure captures exactly what the previous one did, then it is probably unnecessary” [49]. The measurements obtained from a new measurement model should therefore deviate only slightly from existing measurements of the same construct. Moreover, for the model to be viewed as possessing convergent validity, these deviations must be well justified and supported by critical reasoning.
Many value-added models, including the EVAAS MRM, lack convergent validity [2]. For example, in Weapons of Math Destruction [46], O’Neil described Sarah Wysocki, a fifth-grade teacher who received a low score from a value-added model despite excellent reviews from her principal, her colleagues, and her students’ parents.
As another example, measurements of SES obtained from the model described previously and measurements of SES obtained from the National Committee on Vital and Health Statistics would likely correlate somewhat because both operationalizations incorporate income. However, the latter operationalization also incorporates measurements of other observable properties, including wealth, education, occupation, economic pressure, geographic location, and family size [45]. As a result, it is also likely that there would also be significant differences between the two sets of measurements. Investigating these differences might reveal aspects of the substantive nature of SES, such as wealth or education, that are missing from the model described in section 2.2. In other words, and as we described above, assessing convergent validity can reveal qualitative differences between different operationalizations of a construct.
We emphasize that assessing the convergent validity of a measurement model using measurements obtained from measurement models that have not been sufficiently well validated can yield a false sense of security. For example, scores obtained from COMPAS would likely correlate with scores obtained from other models that similarly use arrests as a proxy for crimes committed, thereby obscuring the threat to content validity that we described above.
Discriminant validity
Discriminant validity refers to the extent to which the measurements obtained from a measurement model vary in ways that suggest that the operationalization may be inadvertently capturing aspects of other constructs. Measurements of one construct should only correlate with measurements of another to the extent that those constructs are themselves related. As a special case, if two constructs are totally unrelated, then there should be no correlation between their measurements [25].
Establishing discriminant validity can be especially challenging when a construct has relationships with many other constructs. SES, for example, is related to almost all social and economic constructs, albeit to varying extents. For instance, SES and gender are somewhat related due to labor segregation and the persistent gender wage gap, while SES and race are much more closely related due to historical racial inequalities resulting from structural racism. When assessing the discriminant validity of the model described previously, we would therefore hope to find correlations that reflect these relationships. If, however, we instead found that the resulting measurements were perfectly correlated with gender or uncorrelated with race, this would suggest a lack of discriminant validity.
As another example, Obermeyer et al. found a strong correlation between measurements of patients’ future care needs, operationalized as future care costs, and race [43]. According to their analysis of one model, only 18% of the patients identified for enrollment in highrisk care management programs were Black. This correlation contradicts expectations. Indeed, given the enormous racial health disparities in the U.S., we might even expect to see the opposite pattern. Further investigation by Obermeyer et al. revealed that this threat to discriminant validity was caused by the confounding factor that we described in section 2.5: Black and white patients with comparable past care needs had radically different past care costs—a consequence of structural racism that was then exacerbated by the model.
Predictive validity
Predictive validity refers to the extent to which the measurements obtained from a measurement model are predictive of measurements of any relevant observable properties (and other unobservable theoretical constructs) thought to be related to the construct purported to be measured, but not incorporated into the operationalization. Assessing predictive validity is therefore distinct from out-of-sample prediction [24, 41]. Predictive validity can be assessed using either qualitative or quantitative methods. We note that in contrast to the aspects of construct validity that we discussed above, predictive validity is primarily concerned with the utility of the measurements, not their meaning.
As a simple illustration of predictive validity, taller people generally weigh more than shorter people. Measurements of a person’s height should therefore be somewhat predictive of their weight. Similarly, a person’s SES is related to many observable properties— ranging from purchasing behavior to media appearances—that are not always incorporated into models for measuring SES. Measurements obtained by using income as a proxy for SES would most likely be somewhat predictive of many of these properties, at least for people at the high and low ends of the income distribution.
We note that the relevant observable properties (and other unobservable theoretical constructs) need not be “downstream” of (i.e., thought to be influenced by) the construct. Predictive validity can also be assessed using “upstream” properties and constructs, provided that they are not incorporated into the operationalization. For example, Obermeyer et al. investigated the extent to which measurements of patients’ future care needs, operationalized as future care costs, were predictive of patients’ health statuses (which were not part of the model that they analyzed) [43]. They found that Black and white patients with comparable future care costs did not have comparable health statuses—a threat to predictive validity caused (again) by the confounding factor described previously.
Hypothesis validity
Hypothesis validity refers to the extent to which the measurements obtained from a measurement model support substantively interesting hypotheses about the construct purported to be measured. Much like predictive validity, hypothesis validity is primarily concerned with the utility of the measurements. We note that the main distinction between predictive validity and hypothesis validity hinges on the definition of “substantively interesting hypotheses.” As a result, the distinction is not always clear cut. For example, is the hypothesis “People with higher SES are more likely to be mentioned in the New York Times” sufficiently substantively interesting? Or would it be more appropriate to use the hypothesized relationship to assess predictive validity? For this reason, some traditions merge predictive and hypothesis validity [e.g., 30].
Turning again to the value-added models discussed previously, it is extremely unlikely that the dramatically variable scores obtained from such models would support most substantively interesting hypotheses involving teacher effectiveness, again suggesting a possible mismatch between the theoretical understanding of the construct purported to be measured and its operationalization.
Using income as a proxy for SES would likely support some— though not all—substantively interesting hypotheses involving SES. For example, many social scientists have studied the relationship between SES and health outcomes, demonstrating that people with lower SES tend to have worse health outcomes. Measurements of SES obtained from the model described previously would likely support this hypothesis, albeit with some notable exceptions. For instance, wealthy college students often have low incomes but good access to healthcare. Combined with their young age, this means that they typically have better health outcomes than other people with comparable incomes. Examining these exceptions might reveal aspects of the substantive nature of SES, such as wealth and education, that are missing from the model described previously.
Consequential validity
Consequential validity, the final aspect in our fairness-oriented conceptualization of construct validity, is concerned with identifying and evaluating the consequences of using the measurements obtained from a measurement model, including any societal impacts. Assessing consequential validity often reveals fairness-related harms. Consequential validity was first introduced by Messick, who argued that the consequences of using the measurements obtained from a measurement model are fundamental to establishing construct validity [40]. This is because the values that are reflected in those consequences both derive from and contribute back the theoretical understanding of the construct purported to be measured. In other words, the “measurements both reflect structure in the natural world, and impose structure upon it,” [26]—i.e., the measurements shape the ways that we understand the construct itself. Assessing consequential validity therefore means answering the following questions: How is the world shaped by using the measurements? What world do we wish to live in? If there are contexts in which the consequences of using the measurements would cause us to compromise values that we wish to uphold, then the measurements should not be used in those contexts.
For example, when designing a kitchen, we might use measurements of a person’s standing height to determine the height at which to place their kitchen countertop. However, this may render the countertop inaccessible to them if they use a wheelchair. As another example, because the Universal Credit benefits system described previously assumed that measuring a person’s monthly income by totaling the wages deposited into their account over a single one-month period would yield error-free measurements, many people—especially those with irregular pay schedules— received substantially lower benefits than they were entitled to.
The consequences of using scores obtained from value-added models are well described in the literature on fairness in measurement. Many school districts have used such scores to make decisions about resource distribution and even teachers’ continued employment, often without any way to contest these decisions [2, 3]. In turn, this has caused schools to manipulate their scores and encouraged teachers to “teach to the test,” instead of designing more diverse and substantive curricula [46]. As well as the cases described above in sections 3.1.1 and 3.2.3, in which teachers were fired on the basis of low scores despite evidence suggesting that their scores might be inaccurate, Amrein-Beardsley and Geiger [3] found that EVAAS consistently gave lower scores to teachers at schools with higher proportions of non-white students, students receiving special education services, lower-SES students, and English language learners. Although it is possible that more effective teachers simply chose not to teach at those schools, it is far more likely that these lower scores reflect societal biases and structural inequalities. When scores obtained from value-added models are used to make decisions about resource distribution and teachers’ continued employment, these biases and inequalities are then exacerbated.
The consequences of using scores obtained from COMPAS are also well described in the literature on fairness in computational systems, most notably by Angwin et al. [4], who showed that COMPAS incorrectly scored Black defendants as high risk more often than white defendants, while incorrectly scoring white defendants as low risk more often than Black defendants. By defining recidivism as “a new misdemeanor or felony arrest within two years,” COMPAS fails to account for false arrests or crimes that do not result in arrests. This assumption therefore encodes and exacerbates racist policing practices, leading to the racial disparities uncovered by Angwin et al. Indeed, by using arrests as a proxy for crimes committed, COMPAS can only exacerbate racist policing practices, rather than transcending them [7, 13, 23, 37, 39]. Furthermore, the COMPAS documentation asserts that “the COMPAS risk scales are actuarial risk assessment instruments. Actuarial risk assessment is an objective method of estimating the likelihood of reoffending. An individual’s level of risk is estimated based on known recidivism rates of offenders with similar characteristics” [19]. By describing COMPAS as an “objective method,” Northpointe misrepresents the measurement modeling process, which necessarily involves making assumptions and is thus never objective. Worse yet, the label of objectiveness obscures the organizational, political, societal, and cultural values that are embedded in COMPAS and reflected in its consequences.
Finally, we return to the high-risk care management models described in section 2.5. By operationalizing greatest care needs as greatest care costs, these models fail to account for the fact that patients with comparable past care needs but different access to care will likely have different past care costs. This omission has the greatest impact on Black patients. Indeed, when analyzing one such model, Obermeyer et al. found that only 18% of the patients identified for enrollment were Black [43]. In addition, Obermeyer et al. found that Black and white patients with comparable future care costs did not have comparable health statuses. In other words, these models exacerbate the enormous racial health disparities in the U.S. as a consequence of a seemingly innocuous assumption.
Measurement: The power to create truth*
Because measurement modeling is often skipped over, researchers and practitioners may be inclined to collapse the distinctions between constructs and their operationalizations in how they talk about, think about, and study the concepts in their research question. But collapsing these distinctions removes opportunities to anticipate and mitigate fairness-related harms by eliding the space in which they are most often introduced. Further compounding this issue is the fact that measurements of unobservable theoretical constructs are often treated as if they were obtained directly and without errors—i.e., a source of ground truth. Measurements end up standing in for the constructs purported to be measured, normalizing the assumptions made during the measurement modeling process and embedding them throughout society. In other words, “measures are more than a creation of society, they create society.” [1]. Collapsing the distinctions between constructs and their operationalizations is therefore not just theoretically or pedantically concerning—it is practically concerning with very real, fairness-related consequences.
How we decide to measure what we are researching is influenced by our backgrounds, including our culture, implicit biases, and individual experiences. For me as a middle-class, cisgender white woman, the decisions I make about measurement will probably default to ones that make the most sense to me and others like me, and thus measure characteristics about us most accurately if I don’t think carefully about it. There are major implications for research here because this could affect the validity of my measurements for other populations.
This doesn’t mean that standardized scales or indices, for instance, won’t work for diverse groups of people. What it means is that researchers must not ignore difference in deciding how to measure a variable in their research. Doing so may serve to push already marginalized people further into the margins of academic research and, consequently, social work intervention. Social work researchers, with our strong orientation toward celebrating difference and working for social justice, are obligated to keep this in mind for ourselves and encourage others to think about it in their research, too.
This involves reflecting on what we are measuring, how we are measuring, and why we are measuring. Do we have biases that impacted how we operationalized our concepts? Did we include stakeholders and gatekeepers in the development of our concepts? This can be a way to gain access to vulnerable populations. What feedback did we receive on our measurement process and how was it incorporated into our work? These are all questions we should ask as we are thinking about measurement. Further, engaging in this intentionally reflective process will help us maximize the chances that our measurement will be accurate and as free from bias as possible.
How we decide to measure our variables determines what kind of data we end up with in our research project. Because scientific processes are a part of our sociocultural context, the same biases and oppressions we see in the real world can be manifested or even magnified in research data. Jagadish and colleagues (2021)[1] presents four dimensions of data equity that are relevant to consider: in representation of non-dominant groups within data sets; in how data is collected, analyzed, and combined across datasets; in equitable and participatory access to data, and finally in the outcomes associated with the data collection. Historically, we have mostly focused on the outcomes of measures producing outcomes that are biased in one way or another, and this section reviews many such examples. However, it is important to note that equity must also come from designing measures that respond to questions like:
- Are groups historically suppressed from the data record represented in the sample?
- Are equity data gathered by researchers and used to uncover and quantify inequity?
- Are the data accessible across domains and levels of expertise, and can community members participate in the design, collection, and analysis of the public data record?
- Are the data collected used to monitor and mitigate inequitable impacts?
So, it’s not just about whether measures work for one population for another. Data equity is about the context in which data are created from how we measure people and things. We agree with these authors that data equity should be considered within the context of automated decision-making systems and recognizing a broader literature around the role of administrative systems in creating and reinforcing discrimination. To combat the inequitable processes and outcomes we describe below, researchers must foreground equity as a core component of measurement.
Key Takeaways
- Mismatches between conceptualization and measurement are often places in which bias and systemic injustice enter the research process.
- Measurement modeling is a way of foregrounding researcher’s assumptions in how they connect their conceptual definitions and operational definitions.
- Social work research consumers should critically evaluate the construct validity and reliability of measures in the studies of social work populations.
Exercises
- Examine an article that uses quantitative methods to investigate your topic area.
- Identify the conceptual definitions the authors used.
- These are usually in the introduction section.
- Identify the operational definitions the authors used.
- These are usually in the methods section in a subsection titled measures.
- List the assumptions that link the conceptual and operational definitions.
- For example, that attendance can be measured by a classroom sign-in sheet.
- Do the authors identify any limitations for their operational definitions (measures) in the limitations or methods section?
- Do you identify any limitations in how the authors operationalized their variables?
- Apply the specific subtypes of construct validity and reliability.
10.3 Positionality
Learning Objectives
Learners will be able to…
- Define positionality and explain its impact on the research process
- Identify your positionality using reflexivity
- Reflect on the strengths and limitations of researching as an outsider or insider to the population under study
Measurement modeling is often overlooked because scientists’ expert conceptualizations are assumed to be objectively true. Quantitative methods work best when the researcher’s subjectivity is as far removed from the observation and analysis as possible. This section describes the scientific process of reflexively engaging with your own positionality as a core component of the research process.
Positionality
Student researchers in the social sciences are usually required to identify and articulate their positionality. Frequently teachers and supervisors will expect work to include information about the student’s positionality and its influence on their research. Yet for those commencing a research journey, this may often be difficult and challenging, as students are unlikely to have been required to do so in previous studies. Novice researchers often have difficulty both in identifying exactly what positionality is and in outlining their own. This paper explores researcher positionality and its influence on the research process, so that new researchers may better understand why it is important. Researcher positionality is explained, reflexivity is discussed, and the ‘insider-outsider’ debate is critiqued.
The term positionality both describes an individual’s world view and the position they adopt about a research task and its social and political context (Foote & Bartell 2011, Savin-Baden & Major, 2013 and Rowe, 2014). The individual’s world view or ‘where the researcher is coming from’ concerns ontological assumptions (an individual’s beliefs about the nature of social reality and what is knowable about the world), epistemological assumptions (an individual’s beliefs about the nature of knowledge) and assumptions about human nature and agency (individual’s assumptions about the way we interact with our environment and relate to it) (Sikes, 2004, Bahari, 2010, Scotland, 2012, Ormston, et al. 2014, Marsh, et al. 2018 and Grix, 2019). These are colored by an individual’s values and beliefs that are shaped by their political allegiance, religious faith, gender, sexuality, historical and geographical location, ethnicity, race, social class, and status, (dis) abilities and so on (Sikes, 2004, Wellington, et al. 2005 and Marsh, et al. 2018). Positionality “reflects the position that the researcher has chosen to adopt within a given research study” (Savin-Baden & Major, 2013 p.71, emphasis mine). It influences both how research is conducted, its outcomes, and results (Rowe, 2014). It also influences what a researcher has chosen to investigate in the first place (Malterud, 2001; Grix, 2019).
Positionality is normally identified by locating the researcher about three areas: (1) the subject under investigation, (2) the research participants, and (3) the research context and process (ibid.). Some aspects of positionality are culturally ascribed or generally regarded as being fixed, for example, gender, race, skin-color, nationality. Others, such as political views, personal life-history, and experiences, are more fluid, subjective, and contextual (Chiseri-Strater, 1996). The fixed aspects may predispose someone towards a particular point or point of view, however, that does not mean that these necessarily automatically lead to particular views or perspectives. For example, one may think it would be antithetical for a black African-American to be a member of a white, conservative, right-wing, racist, supremacy group, and, equally, that such a group would not want African-American members. Yet Jansson(2010), in his research on The League of the South, found that not only did a group of this kind have an African-American member, but that he was “warmly welcomed” (ibid. p.21). Mullings (1999, p. 337) suggests that “making the wrong assumptions about the situatedness of an individual’s knowledge based on perceived identity differences may end… access to crucial informants in a research project”. This serves as a reminder that new researchers should not, therefore, make any assumptions about other’s perspectives & world-view and pigeonhole someone based on their own (mis)perceptions of them.
Reflexivity
Positionality requires that both acknowledgment and allowance are made by the researcher to locate their views, values, and beliefs about the research design, conduct, and output(s). Self-reflection and a reflexive approach are both a necessary prerequisite and an ongoing process for the researcher to be able to identify, construct, critique, and articulate their positionality. Simply stated, reflexivity is the concept that researchers should acknowledge and disclose their selves in their research, seeking to understand their part in it, or influence on it (Cohen et al., 2011). Reflexivity informs positionality. It requires an explicit self-consciousness and self-assessment by the researcher about their views and positions and how these might, may, or have, directly or indirectly influenced the design, execution, and interpretation of the research data findings (Greenbank, 2003, May & Perry, 2017). Reflexivity necessarily requires sensitivity by the researcher to their cultural, political, and social context (Bryman, 2016) because the individual’s ethics, personal integrity, and social values, as well as their competency, influence the research process (Greenbank, 2003, Bourke, 2014).
As a way of researchers commencing a reflexive approach to their work Malterud (2001, p.484) suggests that Reflexivity starts by identifying preconceptions brought into the project by the researcher, representing previous personal and professional experiences, pre-study beliefs about how things are and what is to be investigated, motivation and qualifications for exploration of the field, and perspectives and theoretical foundations related to education and interests. It is important for new researchers to note that their values can, frequently, and usually do change over time. As such, the subjective contextual aspects of a researcher’s positionality or ‘situatedness’ change over time (Rowe, 2014). Through using a reflexive approach, researchers should continually be aware that their positionality is never fixed and is always situation and context-dependent. Reflexivity is an essential process for informing developing and shaping positionality, which may clearly articulated.
Positionality impacts the research process
It is essential for new researchers to acknowledge that their positionality is unique to them and that it can impact all aspects and stages of the research process. As Foote and Bartell (2011, p.46) identify “The positionality that researchers bring to their work, and the personal experiences through which positionality is shaped, may influence what researchers may bring to research encounters, their choice of processes, and their interpretation of outcomes.” Positionality, therefore, can be seen to affect the totality of the research process. It acknowledges and recognizes that researchers are part of the social world they are researching and that this world has already been interpreted by existing social actors. This is the opposite of a positivistic conception of objective reality (Cohen et al., 2011; Grix, 2019). Positionality implies that the social-historical-political location of a researcher influences their orientations, i.e., that they are not separate from the social processes they study.
Simply stated, there is no way we can escape the social world we live in to study it (Hammersley & Atkinson, 1995; Malterud, 2001). The use of a reflexive approach to inform positionality is a rejection of the idea that social research is separate from wider society and the individual researcher’s biography. A reflexive approach suggests that, rather than trying to eliminate their effect, researchers should acknowledge and disclose their selves in their work, aiming to understand their influence on and in the research process. It is important for new researchers to note here that their positionality not only shapes their work but influences their interpretation, understanding, and, ultimately, their belief in the truthfulness and validity of other’s research that they read or are exposed to. It also influences the importance given to, the extent of belief in, and their understanding of the concept of positionality.
Open and honest disclosure and exposition of positionality should show where and how the researcher believes that they have, or may have, influenced their research. The reader should then be able to make a better-informed judgment as to the researcher’s influence on the research process and how ‘truthful’ they feel the research data is. Sikes (2004, p.15) argues that It is important for all researchers to spend some time thinking about how they are paradigmatically and philosophically positioned and for them to be aware of how their positioning -and the fundamental assumptions they hold might influence their research related thinking in practice. This is about being a reflexive and reflective and, therefore, a rigorous researcher who can present their findings and interpretations in the confidence that they have thought about, acknowledged and been honest and explicit about their stance and the influence it has had upon their work. For new researchers doing this can be a complex, difficult, and sometimes extremely time-consuming process. Yet, it is essential to do so. Sultana (2007, p.380), for example, argues that it is “critical to pay attention to positionality, reflexivity, the production of knowledge… to undertake ethical research”. The clear implication being that, without reflexivity on the part of the researcher, their research may not be conducted ethically. Given that no contemporary researcher should engage in unethical research (BERA, 2018), reflexivity and clarification of one’s positionality may, therefore, be seen as essential aspects of the research process.
Finding your positionality
Savin-Baden & Major (2013) identify three primary ways that a researcher may identify and develop their positionality.
- Firstly, locating themselves about the subject (i.e., acknowledging personal positions that have the potential to influence the research.)
- Secondly, locating themselves about the participants (i.e., researchers individually considering how they view themselves, as well as how others view them, while at the same time acknowledging that as individuals they may not be fully aware of how they and others have constructed their identities, and recognizing that it may not be possible to do this without considered in-depth thought and critical analysis.)
- Thirdly, locating themselves about the research context and process. (i.e., acknowledging that research will necessarily be influenced by themselves and by the research context.
- To those, I would add a fourth component; that of time. Investigating and clarifying one’s positionality takes time. New researchers should recognize that exploring their positionality and writing a positionality statement can take considerable time and much ‘soul searching’. It is not a process that can be rushed.
Engaging in a reflexive approach should allow for a reduction of bias and partisanship (Rowe, 2014). However, it must be acknowledged by novice researchers that, no matter how reflexive they are, they can never objectively describe something as it is. We can never objectively describe reality (Dubois, 2015). It must also be borne in mind that language is a human social construct. Experiences and interpretations of language are individually constructed, and the meaning of words is individually and subjectively constructed (von-Glaserfield, 1988). Therefore, no matter how much reflexive practice a researcher engages in, there will always still be some form of bias or subjectivity. Yet, through exploring their positionality, the novice researcher increasingly becomes aware of areas where they may have potential bias and, over time, are better able to identify these so that they may then take account of them. (Ormston et al., 2014) suggest that researchers should aim to achieve ‘empathetic neutrality,’ i.e., that they should Strive to avoid obvious, conscious, or systematic bias and to be as neutral as possible in the collection, interpretation, and presentation of data…[while recognizing that] this aspiration can never be fully attained – all research will be influenced by the researcher and there is no completely ‘neutral’ or ‘objective’ knowledge.
Positionality statements
Regardless of how they are positioned in terms of their epistemological assumptions, it is crucial that researchers are clear in their minds as to the implications of their stance, that they state their position explicitly (Sikes, 2004). Positionality is often formally expressed in research papers, masters-level dissertations, and doctoral theses via a positionality statement, essentially an explanation of how the researcher developed and how they became the researcher they are then. For most people, this will necessarily be a fluid statement that changes as they develop both through conducting a specific research project and throughout their research career.
A strong positionality statement will typically include a description of the researcher’s lenses (such as their philosophical, personal, theoretical beliefs and perspective through which they view the research process), potential influences on the research (such as age, political beliefs, social class, race, ethnicity, gender, religious beliefs, previous career), the researcher’s chosen or pre-determined position about the participants in the project (e.g., as an insider or an outsider), the research-project context and an explanation as to how, where, when and in what way these might, may, or have, influenced the research process (Savin-Baden & Major, 2013). Producing a good positionality statement takes time, considerable thought, and critical reflection. It is particularly important for novice researchers to adopt a reflexive approach and recognize that “The inclusion of reflective accounts and the acknowledgment that educational research cannot be value-free should be included in all forms of research” (Greenbank, 2003).
Yet new researchers also need to realize that reflexivity is not a panacea that eradicates the need for awareness of the limits of self-reflexivity. Reflexivity can help to clarify and contextualize one’s position about the research process for both the researcher, the research participants, and readers of research outputs. Yet, it is not a guarantee of more honest, truthful, or ethical research. Nor is it a guarantee of good research (Delamont, 2018). No matter how critically reflective and reflexive one is, aspects of the self can be missed, not known, or deliberately hidden, see, for example, Luft and Ingham’s (1955) Johari Window – the ‘blind area’ known to others but not to oneself and the ‘hidden area,’ not known to others and not known to oneself. There are always areas of ourselves that we are not aware of, areas that only other people are aware of, and areas that no one is aware of. One may also, particularly in the early stages of reflection, not be as honest with one’s self as one needs to be (Holmes, 2019).
Novice researchers should realize that, right from the very start of the research process, that their positionality will affect their research and will impact Son their understanding, interpretation, acceptance, and belief, or non-acceptance and disbelief of other’s research findings. It will also influence their views about reflexivity and the relevance and usefulness of adopting a reflexive approach and articulating their positionality. Each researcher’s positionality affects the research process, and their outputs as well as their interpretation of other’s research. (Smith, 1999) neatly sums this up, suggesting that “Objectivity, authority and validity of knowledge is challenged as the researcher’s positionality… is inseparable from the research findings”.
Do you need lived experience to research a topic?
The position of the researcher as being an insider or an outsider to the culture being studied and, both, whether one position provides the researcher with an advantageous position compared with the other, and its effect on the research process (Hammersley 1993 and Weiner et al. 2012) has been, and remains, a key debate. One area of contention regarding the insider outsider debate is whether or not being an insider to the culture positions the researcher more, or less, advantageously than an outsider. Epistemologically this is concerned with whether and how it is possible to present information accurately and truthfully.
Merton’s long-standing definition of insiders and outsiders is that “Insiders are the members of specified groups and collectives or occupants of specified social statuses: Outsiders are non-members” (Merton, 1972). Others identify the insider as someone whose personal biography (gender, race, skin-color, class, sexual orientation and so on) gives them a ‘lived familiarity’ with and a priori knowledge of the group being researched. At the same time, the outsider is a person/researcher who does not have any prior intimate knowledge of the group being researched (Griffith, 1998, cited in Mercer, 2007). There are various lines of the argument put forward to emphasize the advantages and disadvantages of each position. In its simplest articulation, the insider perspective essentially questions the ability of outsider scholars to competently understand the experiences of those inside the culture, while the outsider perspective questions the ability of the insider scholar to sufficiently detach themselves from the culture to be able to study it without bias (Kusow, 2003).
For a more extensive discussion, see (Merton, 1972). The main arguments are outlined below. Advantages of an insider position include:
- (1) easier access to the culture being studied, as the researcher is regarded as being ‘one of us’ (Sanghera & Bjokert 2008),
- (2) the ability to ask more meaningful or insightful questions (due to possession of a priori knowledge),
- (3) the researcher may be more trusted so may secure more honest answers,
- (4) the ability to produce a more truthful, authentic or ‘thick’ description (Geertz, 1973) and understanding of the culture,
- (5) potential disorientation due to ‘culture shock’ is removed or reduced, and
- (6) the researcher is better able to understand the language, including colloquial language, and non-verbal cues.
Disadvantages of an insider position include:
- (1) the researcher may be inherently and unknowingly biased, or overly sympathetic to the culture,
- (2) they may be too close to and familiar with the culture (a myopic view), or bound by custom and code so that they are unable to raise provocative or taboo questions,
- (3) research participants may assume that because the insider is ‘one of us’ that they possess more or better insider knowledge than they do, (which they may not) and that their understandings are the same (which they may not be). Therefore information which should be ‘obvious’ to the insider, may not be articulated or explained,
- (4) an inability to bring an external perspective to the process,
- (5) ‘dumb’ questions which an outsider may legitimately ask, may not be able to be asked (Naaek et al. 2010), and
- (6) respondents may be less willing to reveal sensitive information than they would be to an outsider who they will have no future contact with.
Unfortunately, it is the case that each of the above advantages can, depending upon one’s perspective, be equally viewed as being disadvantages, and each of the disadvantages as being advantages, so that “The insider’s strengths become the outsider’s weaknesses and vice versa” (Merriam et al., 2001, p.411). Whether either position offers an advantage over the other is questionable. (Hammersley 1993) for example, argues that there are “No overwhelming advantages to being an insider or outside” but that each position has both advantages and disadvantages, which take on slightly different weights depending on the specific circumstances and the purpose of the research. Similarly, Mercer (2007) suggests that it is a ‘double-edged sword’ in that what is gained in one area may be lost in another, for example, detailed insider knowledge may mean that the ‘bigger picture’ is not seen.
There is also an argument that insider or outsider as opposites may be an artificial construct. There may be no clear dichotomy between the two positions (Herod, 1999), the researcher may not be either an insider or an outsider, but the positions can be seen as a continuum with conceptual rather than actual endpoints (Christensen & Dahl, 1997, cited in Mercer, 2007). Similarly, Mercer (ibid. p.1) suggests that The insider/outsider dichotomy is, in reality, a continuum with multiple dimensions and that all researchers constantly move back and forth along several axes, depending upon time, location, participants, and topic. I would argue that a researcher may inhabit multiple positions along that continuum at the same time. Merton (1972, p.28) argues that Sociologically speaking, there is nothing fixed about the boundaries separating Insiders from Outsiders. As situations involving different values arise, different statuses are activated, and the lines of separation shift. Traditionally emic and etic perspectives are “Often seen as being at odds – as incommensurable paradigms” (Morris et al. 1999 p.781). Yet the insider and outsider roles are essentially products of the particular situation in which research takes place (Kusow, 2003). As such, they are both researcher and context-specific, with no clearly -cut boundaries. And as such may not be a divided binary (Mullings, 1999, Chacko, 2004). Researchers may straddle both positions; they may be simultaneously and insider and an outsider (Mohammed, 2001).
For example, a mature female Saudi Ph.D. student studying social work may be an insider by being a student, yet as a doctoral student, an outsider to undergraduates. They may be regarded as being an insider by Saudi students, but an outsider by students from other countries; an insider to female students, but an outsider to male students; an insider to Muslim students, an outsider to Christian students; an insider to mature students, an outsider to younger students, and so on. Combine these with the many other insider-outsider positions, and it soon becomes clear that it is rarely a case of simply being an insider or outsider, but that of the researcher simultaneously residing in several positions. If insiderness is interpreted by the researcher as implying a single fixed status (such as sex, race, religion, etc.), then the terms insider and outsider are more likely to be seen by them as dichotomous, (because, for example, a person cannot be simultaneously both male and female, black and white, Christian and Muslim). If, on the other hand, a more pluralistic lens is used, accepting that human beings cannot be classified according to a single ascribed status, then the two terms are likely to be considered as being poles of a continuum (Mercer, 2007). The implication is that, as part of the process of reflexivity and articulating their positionality, novice researchers should consider how they perceive the concept of insider-outsiderness– as a continuum or a dichotomy, and take this into account. It has been suggested (e.g., Ritchie, et al. 2009, Kirstetter, 2012) that recent qualitative research has seen a blurring of the separation between insiderness and outsiderness and that it may be more appropriate to define a researcher’s stance by their physical and psychological distance from the research phenomenon under study rather than their paradigmatic position.
Key Takeaways
- Positionality is integral to the process of qualitative research, as is the researcher’s awareness of the lack of stasis of our own and other’s positionality
- identifying and clearly articulating your positionality in respect of the project being undertaken may not be a simple or quick process, yet it is essential to do so.
- Pay particular attention to your multiple positions as an insider or outsider to the research participants and setting(s) where the work is conducted, acknowledging there may be both advantages and disadvantages that may have far-reaching implications for the process of data gathering and interpretation.
- While engaging in reflexive practice and articulating their positionality is not a guarantee of higher quality research, that through doing so, you will become a better researcher.
Exercises
- What is your relationship to the population in your study? (insider, outsider, both)
- How is your perspective on the topic informed by your lived experience?
- Any biases, beliefs, etc. that might influence you?
- Why do you want to answer your working question? (i.e., what is your research project’s aim)
Go to Google News, YouTube or TikTok, or an internet search engine, and look for first-person narratives about your topic. Try to look for sources that include the person’s own voice through quotations or video/audio recordings.
- How is your perspective on the topic different from the person in your narrative?’
- How do those differences relate to positionality?
- Look at a research article on your topic.
- How might the study have been different if the person in your narrative were part of the research team?
- What differences might there be in ethics, sampling, measures, or design?
10.4 Post-positivism: The assumptions of quantitative methods
Learning Objectives
Learners will be able to…
- Ground your research project and working question in the philosophical assumptions of social science
- Define the terms ‘ontology‘ and ‘epistemology‘ and explain how they relate to quantitative and qualitative research methods
- Apply feminist, anti-racist, and decolonization critiques of social science to your project
- Define axiology and describe the axiological assumptions of research projects
Measurement modeling itself relies on a number of underlying assumptions about truth, discovery, and power that shape the research process. These assumptions are easy to overlook, but they are crucial for understanding how quantitative methods have adapted over time to become more robust against invalid, unreliable, and unfair measures.
What are your assumptions?
Social workers must understand measurement theory to engage in social justice work. That’s because measurement theory and its supporting philosophical assumptions will help sharpen your perceptions of the social world. They help social workers build heuristics that can help identify the fundamental assumptions at the heart of social conflict and social problems. They alert you to the patterns in the underlying assumptions that different people make and how those assumptions shape their worldview, what they view as true, and what they hope to accomplish. In the next section, we will review feminist and other critical perspectives on research, and they should help inform you of how assumptions about research can reinforce oppression.
Understanding these deeper structures behind research evidence is a true gift of social work research. Because we acknowledge the usefulness and truth value of multiple philosophies and worldviews contained in this chapter, we can arrive at a deeper and more nuanced understanding of the social world.
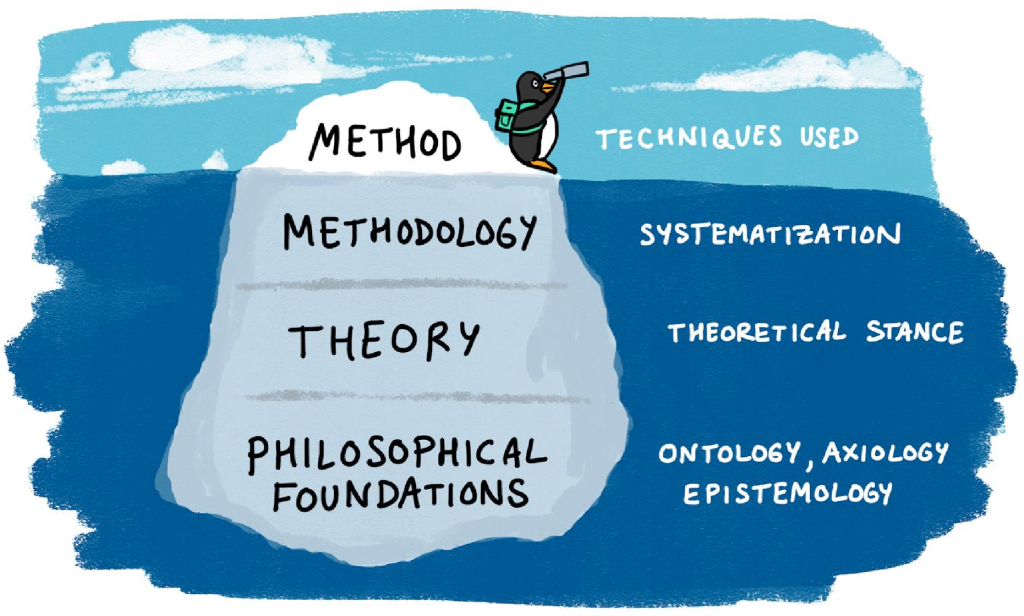
Building your ice float
Before we can dive into philosophy, we need to recall out conversation from Chapter 1 about objective truth and subjective truths. Let’s test your knowledge with a quick example. Is crime on the rise in the United States? A recent Five Thirty Eight article highlights the disparity between historical trends on crime that are at or near their lowest in the thirty years with broad perceptions by the public that crime is on the rise (Koerth & Thomson-DeVeaux, 2020).[8] Social workers skilled at research can marshal objective truth through statistics, much like the authors do, to demonstrate that people’s perceptions are not based on a rational interpretation of the world. Of course, that is not where our work ends. Subjective truths might decenter this narrative of ever-increasing crime, deconstruct its racist and oppressive origins, or simply document how that narrative shapes how individuals and communities conceptualize their world.
Objective does not mean right, and subjective does not mean wrong. Researchers must understand what kind of truth they are searching for so they can choose a theoretical framework, methodology, and research question that matches. As we discussed in Chapter 1, researchers seeking objective truth (one of the philosophical foundations at the bottom of Figure 7.1) often employ quantitative methods (one of the methods at the top of Figure 7.1). Similarly, researchers seeking subjective truths (again, at the bottom of Figure 7.1) often employ qualitative methods (at the top of Figure 7.1). This chapter is about the connective tissue, and by the time you are done reading, you should have a first draft of a theoretical and philosophical (a.k.a. paradigmatic) framework for your study.
Ontology: Assumptions about what is real & true
In section 1.2, we reviewed the two types of truth that social work researchers seek—objective truth and subjective truths —and linked these with the methods—quantitative and qualitative—that researchers use to study the world. If those ideas aren’t fresh in your mind, you may want to navigate back to that section for an introduction.
These two types of truth rely on different assumptions about what is real in the social world—i.e., they have a different ontology. Ontology refers to the study of being (literally, it means “rational discourse about being”). In philosophy, basic questions about existence are typically posed as ontological, e.g.:
- What is there?
- What types of things are there?
- How can we describe existence?
- What kind of categories can things go into?
- Are the categories of existence hierarchical?
Objective vs. subjective ontologies
At first, it may seem silly to question whether the phenomena we encounter in the social world are real. Of course you exist, your thoughts exist, your computer exists, and your friends exist. You can see them with your eyes. This is the ontological framework of realism, which simply means that the concepts we talk about in science exist independent of observation (Burrell & Morgan, 1979).[9] Obviously, when we close our eyes, the universe does not disappear. You may be familiar with the philosophical conundrum: “If a tree falls in a forest and no one is around to hear it, does it make a sound?”
The natural sciences, like physics and biology, also generally rely on the assumption of realism. Lone trees falling make a sound. We assume that gravity and the rest of physics are there, even when no one is there to observe them. Mitochondria are easy to spot with a powerful microscope, and we can observe and theorize about their function in a cell. The gravitational force is invisible, but clearly apparent from observable facts, such as watching an apple fall from a tree. Of course, out theories about gravity have changed over the years. Improvements were made when observations could not be correctly explained using existing theories and new theories emerged that provided a better explanation of the data.
As we discussed in section 1.2, culture-bound syndromes are an excellent example of where you might come to question realism. Of course, from a Western perspective as researchers in the United States, we think that the Diagnostic and Statistical Manual (DSM) classification of mental health disorders is real and that these culture-bound syndromes are aberrations from the norm. But what about if you were a person from Korea experiencing Hwabyeong? Wouldn’t you consider the Western diagnosis of somatization disorder to be incorrect or incomplete? This conflict raises the question–do either Hwabyeong or DSM diagnoses like post-traumatic stress disorder (PTSD) really exist at all…or are they just social constructs that only exist in our minds?
If your answer is “no, they do not exist,” you are adopting the ontology of anti-realism (or relativism), or the idea that social concepts do not exist outside of human thought. Unlike the realists who seek a single, universal truth, the anti-realists perceive a sea of truths, created and shared within a social and cultural context. Unlike objective truth, which is true for all, subjective truths will vary based on who you are observing and the context in which you are observing them. The beliefs, opinions, and preferences of people are actually truths that social scientists measure and describe. Additionally, subjective truths do not exist independent of human observation because they are the product of the human mind. We negotiate what is true in the social world through language, arriving at a consensus and engaging in debate within our socio-cultural context.
These theoretical assumptions should sound familiar if you’ve studied social constructivism or symbolic interactionism in your other MSW courses, most likely in human behavior in the social environment (HBSE).[10] From an anti-realist perspective, what distinguishes the social sciences from natural sciences is human thought. When we try to conceptualize trauma from an anti-realist perspective, we must pay attention to the feelings, opinions, and stories in people’s minds. In their most radical formulations, anti-realists propose that these feelings and stories are all that truly exist.
What happens when a situation is incorrectly interpreted? Certainly, who is correct about what is a bit subjective. It depends on who you ask. Even if you can determine whether a person is actually incorrect, they think they are right. Thus, what may not be objectively true for everyone is nevertheless true to the individual interpreting the situation. Furthermore, they act on the assumption that they are right. We all do. Much of our behaviors and interactions are a manifestation of our personal subjective truth. In this sense, even incorrect interpretations are truths, even though they are true only to one person or a group of misinformed people. This leads us to question whether the social concepts we think about really exist. For researchers using subjective ontologies, they might only exist in our minds; whereas, researchers who use objective ontologies which assume these concepts exist independent of thought.
How do we resolve this dichotomy? As social workers, we know that often times what appears to be an either/or situation is actually a both/and situation. Let’s take the example of trauma. There is clearly an objective thing called trauma. We can draw out objective facts about trauma and how it interacts with other concepts in the social world such as family relationships and mental health. However, that understanding is always bound within a specific cultural and historical context. Moreover, each person’s individual experience and conceptualization of trauma is also true. Much like a client who tells you their truth through their stories and reflections, when a participant in a research study tells you what their trauma means to them, it is real even though only they experience and know it that way. By using both objective and subjective analytic lenses, we can explore different aspects of trauma—what it means to everyone, always, everywhere, and what is means to one person or group of people, in a specific place and time.
Epistemology: Assumptions about how we know things
Having discussed what is true, we can proceed to the next natural question—how can we come to know what is real and true? This is epistemology. Epistemology is derived from the Ancient Greek epistēmē which refers to systematic or reliable knowledge (as opposed to doxa, or “belief”). Basically, it means “rational discourse about knowledge,” and the focus is the study of knowledge and methods used to generate knowledge. Epistemology has a history as long as philosophy, and lies at the foundation of both scientific and philosophical knowledge.
Epistemological questions include:
- What is knowledge?
- How can we claim to know anything at all?
- What does it mean to know something?
- What makes a belief justified?
- What is the relationship between the knower and what can be known?
While these philosophical questions can seem far removed from real-world interaction, thinking about these kinds of questions in the context of research helps you target your inquiry by informing your methods and helping you revise your working question. Epistemology is closely connected to method as they are both concerned with how to create and validate knowledge. Research methods are essentially epistemologies – by following a certain process we support our claim to know about the things we have been researching. Inappropriate or poorly followed methods can undermine claims to have produced new knowledge or discovered a new truth. This can have implications for future studies that build on the data and/or conceptual framework used.
Research methods can be thought of as essentially stripped down, purpose-specific epistemologies. The knowledge claims that underlie the results of surveys, focus groups, and other common research designs ultimately rest on epistemological assumptions of their methods. Focus groups and other qualitative methods usually rely on subjective epistemological (and ontological) assumptions. Surveys and and other quantitative methods usually rely on objective epistemological assumptions. These epistemological assumptions often entail congruent subjective or objective ontological assumptions about the ultimate questions about reality.
Objective vs. subjective epistemologies
One key consideration here is the status of ‘truth’ within a particular epistemology or research method. If, for instance, some approaches emphasize subjective knowledge and deny the possibility of an objective truth, what does this mean for choosing a research method?
We began to answer this question in Chapter 1 when we described the scientific method and objective and subjective truths. Epistemological subjectivism focuses on what people think and feel about a situation, while epistemological objectivism focuses on objective facts irrelevant to our interpretation of a situation (Lin, 2015).[11]
While there are many important questions about epistemology to ask (e.g., “How can I be sure of what I know?” or “What can I not know?” see Willis, 2007[12] for more), from a pragmatic perspective most relevant epistemological question in the social sciences is whether truth is better accessed using numerical data or words and performances. Generally, scientists approaching research with an objective epistemology (and realist ontology) will use quantitative methods to arrive at scientific truth. Quantitative methods examine numerical data to precisely describe and predict elements of the social world. For example, while people can have different definitions for poverty, an objective measurement such as an annual income of “less than $25,100 for a family of four” provides a precise measurement that can be compared to incomes from all other people in any society from any time period, and refers to real quantities of money that exist in the world. Mathematical relationships are uniquely useful in that they allow comparisons across individuals as well as time and space. In this book, we will review the most common designs used in quantitative research: surveys and experiments. These types of studies usually rely on the epistemological assumption that mathematics can represent the phenomena and relationships we observe in the social world.
Although mathematical relationships are useful, they are limited in what they can tell you. While you can learn use quantitative methods to measure individuals’ experiences and thought processes, you will miss the story behind the numbers. To analyze stories scientifically, we need to examine their expression in interviews, journal entries, performances, and other cultural artifacts using qualitative methods. Because social science studies human interaction and the reality we all create and share in our heads, subjectivists focus on language and other ways we communicate our inner experience. Qualitative methods allow us to scientifically investigate language and other forms of expression—to pursue research questions that explore the words people write and speak. This is consistent with epistemological subjectivism’s focus on individual and shared experiences, interpretations, and stories.
It is important to note that qualitative methods are entirely compatible with seeking objective truth. Approaching qualitative analysis with a more objective perspective, we look simply at what was said and examine its surface-level meaning. If a person says they brought their kids to school that day, then that is what is true. A researcher seeking subjective truth may focus on how the person says the words—their tone of voice, facial expressions, metaphors, and so forth. By focusing on these things, the researcher can understand what it meant to the person to say they dropped their kids off at school. Perhaps in describing dropping their children off at school, the person thought of their parents doing the same thing or tried to understand why their kid didn’t wave back to them as they left the car. In this way, subjective truths are deeper, more personalized, and difficult to generalize.
Self-determination and free will
When scientists observe social phenomena, they often take the perspective of determinism, meaning that what is seen is the result of processes that occurred earlier in time (i.e., cause and effect). This process is represented in the classical formulation of a research question which asks “what is the relationship between X (cause) and Y (effect)?” By framing a research question in such a way, the scientist is disregarding any reciprocal influence that Y has on X. Moreover, the scientist also excludes human agency from the equation. It is simply that a cause will necessitate an effect. For example, a researcher might find that few people living in neighborhoods with higher rates of poverty graduate from high school, and thus conclude that poverty causes adolescents to drop out of school. This conclusion, however, does not address the story behind the numbers. Each person who is counted as graduating or dropping out has a unique story of why they made the choices they did. Perhaps they had a mentor or parent that helped them succeed. Perhaps they faced the choice between employment to support family members or continuing in school.
For this reason, determinism is critiqued as reductionistic in the social sciences because people have agency over their actions. This is unlike the natural sciences like physics. While a table isn’t aware of the friction it has with the floor, parents and children are likely aware of the friction in their relationships and act based on how they interpret that conflict. The opposite of determinism is free will, that humans can choose how they act and their behavior and thoughts are not solely determined by what happened prior in a neat, cause-and-effect relationship. Researchers adopting a perspective of free will view the process of, continuing with our education example, seeking higher education as the result of a number of mutually influencing forces and the spontaneous and implicit processes of human thought. For these researchers, the picture painted by determinism is too simplistic.
A similar dichotomy can be found in the debate between individualism and holism. When you hear something like “the disease model of addiction leads to policies that pathologize and oppress people who use drugs,” the speaker is making a methodologically holistic argument. They are making a claim that abstract social forces (the disease model, policies) can cause things to change. A methodological individualist would critique this argument by saying that the disease model of addiction doesn’t actually cause anything by itself. From this perspective, it is the individuals, rather than any abstract social force, who oppress people who use drugs. The disease model itself doesn’t cause anything to change; the individuals who follow the precepts of the disease model are the agents who actually oppress people in reality. To an individualist, all social phenomena are the result of individual human action and agency. To a holist, social forces can determine outcomes for individuals without individuals playing a causal role, undercutting free will and research projects that seek to maximize human agency.
Exercises
- Examine an article from your literature review
- Is human action, or free will, informing how the authors think about the people in their study?
- Or are humans more passive and what happens to them more determined by the social forces that influence their life?
- Reflect on how this project’s assumptions may differ from your own assumptions about free will and determinism. For example, my beliefs about self-determination and free will always inform my social work practice. However, my working question and research project may rely on social theories that are deterministic and do not address human agency.
Radical change
Another assumption scientists make is around the nature of the social world. Is it an orderly place that remains relatively stable over time? Or is it a place of constant change and conflict? The view of the social world as an orderly place can help a researcher describe how things fit together to create a cohesive whole. For example, systems theory can help you understand how different systems interact with and influence one another, drawing energy from one place to another through an interconnected network with a tendency towards homeostasis. This is a more consensus-focused and status-quo-oriented perspective. Yet, this view of the social world cannot adequately explain the radical shifts and revolutions that occur. It also leaves little room for human action and free will. In this more radical space, change consists of the fundamental assumptions about how the social world works.
For example, at the time of this writing, protests are taking place across the world to remember the killing of George Floyd by Minneapolis police and other victims of police violence and systematic racism. Public support of Black Lives Matter, an anti-racist activist group that focuses on police violence and criminal justice reform, has experienced a radical shift in public support in just two weeks since the killing, equivalent to the previous 21 months of advocacy and social movement organizing (Cohn & Quealy, 2020).[13] Abolition of police and prisons, once a fringe idea, has moved into the conversation about remaking the criminal justice system from the ground-up, centering its historic and current role as an oppressive system for Black Americans. Seemingly overnight, reducing the money spent on police and giving that money to social services became a moderate political position.
A researcher centering change may choose to understand this transformation or even incorporate radical anti-racist ideas into the design and methods of their study. For an example of how to do so, see this participatory action research study working with Black and Latino youth (Bautista et al., 2013).[14] Contrastingly, a researcher centering consensus and the status quo might focus on incremental changes what people currently think about the topic. For example, see this survey of social work student attitudes on poverty and race that seeks to understand the status quo of student attitudes and suggest small changes that might change things for the better (Constance-Huggins et al., 2020).[15] To be clear, both studies contribute to racial justice. However, you can see by examining the methods section of each article how the participatory action research article addresses power and values as a core part of their research design, qualitative ethnography and deep observation over many years, in ways that privilege the voice of people with the least power. In this way, it seeks to rectify the epistemic injustice of excluding and oversimplifying Black and Latino youth. Contrast this more radical approach with the more traditional approach taken in the second article, in which they measured student attitudes using a survey developed by researchers.
Exercises
- Examine an article from your literature review
- Traditional studies will be less participatory. The researcher will determine the research question, how to measure it, data collection, etc.
- Radical studies will be more participatory. The researcher seek to undermine power imbalances at each stage of the research process.
- Pragmatically, more participatory studies take longer to complete and are less suited to projects that need to be completed in a short time frame.
Axiology: Assumptions about values
Axiology is the study of values and value judgements (literally “rational discourse about values [a xía]”). In philosophy this field is subdivided into ethics (the study of morality) and aesthetics (the study of beauty, taste and judgement). For the hard-nosed scientist, the relevance of axiology might not be obvious. After all, what difference do one’s feelings make for the data collected? Don’t we spend a long time trying to teach researchers to be objective and remove their values from the scientific method?
Like ontology and epistemology, the import of axiology is typically built into research projects and exists “below the surface”. You might not consciously engage with values in a research project, but they are still there. Similarly, you might not hear many researchers refer to their axiological commitments but they might well talk about their values and ethics, their positionality, or a commitment to social justice.
Our values focus and motivate our research. These values could include a commitment to scientific rigor, or to always act ethically as a researcher. At a more general level we might ask: What matters? Why do research at all? How does it contribute to human wellbeing? Almost all research projects are grounded in trying to answer a question that matters or has consequences. Some research projects are even explicit in their intention to improve things rather than observe them. This is most closely associated with “critical” approaches.
Critical and radical views of science focus on how to spread knowledge and information in a way that combats oppression. These questions are central for creating research projects that fight against the objective structures of oppression—like unequal pay—and their subjective counterparts in the mind—like internalized sexism. For example, a more critical research project would fight not only against statutes of limitations for sexual assault but on how women have internalized rape culture as well. Its explicit goal would be to fight oppression and to inform practice on women’s liberation. For this reason, creating change is baked into the research questions and methods used in more critical and radical research projects.
As part of studying radical change and oppression, we are likely employing a model of science that puts values front-and-center within a research project. All social work research is values-driven, as we are a values-driven profession. Historically, though, most social scientists have argued for values-free science. Scientists agree that science helps human progress, but they hold that researchers should remain as objective as possible—which means putting aside politics and personal values that might bias their results, similar to the cognitive biases we discussed in section 1.1. Over the course of last century, this perspective was challenged by scientists who approached research from an explicitly political and values-driven perspective. As we discussed earlier in this section, feminist critiques strive to understand how sexism biases research questions, samples, measures, and conclusions, while decolonization critiques try to de-center the Western perspective of science and truth.
Linking axiology, epistemology, and ontology
It is important to note that both values-central and values-neutral perspectives are useful in furthering social justice. Values-neutral science is helpful at predicting phenomena. Indeed, it matches well with objectivist ontologies and epistemologies. Let’s examine a measure of depression, the Patient Health Questionnaire (PSQ-9). The authors of this measure spent years creating a measure that accurately and reliably measures the concept of depression. This measure is assumed to measure depression in any person, and scales like this are often translated into other languages (and subsequently validated) for more widespread use . The goal is to measure depression in a valid and reliable manner. We can use this objective measure to predict relationships with other risk and protective factors, such as substance use or poverty, as well as evaluate the impact of evidence-based treatments for depression like narrative therapy.
While measures like the PSQ-9 help with prediction, they do not allow you to understand an individual person’s experience of depression. To do so, you need to listen to their stories and how they make sense of the world. The goal of understanding isn’t to predict what will happen next, but to empathically connect with the person and truly understand what’s happening from their perspective. Understanding fits best in subjectivist epistemologies and ontologies, as they allow for multiple truths (i.e. that multiple interpretations of the same situation are valid). Although all researchers addressing depression are working towards socially just ends, the values commitments researchers make as part of the research process influence them to adopt objective or subjective ontologies and epistemologies.
Exercises
What role will values play in your study?
- Are you looking to be as objective as possible, putting aside your own values?
- Or are you infusing values into each aspect of your research design?
Remember that although social work is a values-based profession, that does not mean that all social work research is values-informed. The majority of social work research is objective and tries to be value-neutral in how it approaches research.
Positivism: Researcher as “expert”
Positivism (and post-positivism) is the dominant paradigm in social science. We define paradigm a set of common philosophical (ontological, epistemological, and axiological) assumptions that inform research. The four paradigms we describe in this section refer to patterns in how groups of researchers resolve philosophical questions. Some assumptions naturally make sense together, and paradigms grow out of researchers with shared assumptions about what is important and how to study it. Paradigms are like “analytic lenses” and a provide framework on top of which we can build theoretical and empirical knowledge (Kuhn, 1962).[16] Consider this video of an interview with world-famous physicist Richard Feynman in which he explains why “when you explain a ‘why,’ you have to be in some framework that you allow something to be true. Otherwise, you are perpetually asking why.” In order to answer basic physics question like “what is happening when two magnets attract?” or a social work research question like “what is the impact of this therapeutic intervention on depression,” you must understand the assumptions you are making about social science and the social world. Paradigmatic assumptions about objective and subjective truth support methodological choices like whether to conduct interviews or send out surveys, for example.
When you think of science, you are probably thinking of positivistic science–like the kind the physicist Richard Feynman did. It has its roots in the scientific revolution of the Enlightenment. Positivism is based on the idea that we can come to know facts about the natural world through our experiences of it. The processes that support this are the logical and analytic classification and systemization of these experiences. Through this process of empirical analysis, Positivists aim to arrive at descriptions of law-like relationships and mechanisms that govern the world we experience.
Positivists have traditionally claimed that the only authentic knowledge we have of the world is empirical and scientific. Essentially, positivism downplays any gap between our experiences of the world and the way the world really is; instead, positivism determines objective “facts” through the correct methodological combination of observation and analysis. Data collection methods typically include quantitative measurement, which is supposed to overcome the individual biases of the researcher.
Positivism aspires to high standards of validity and reliability supported by evidence, and has been applied extensively in both physical and social sciences. Its goal is familiar to all students of science: iteratively expanding the evidence base of what we know is true. We can know our observations and analysis describe real world phenomena because researchers separate themselves and objectively observe the world, placing a deep epistemological separation between “the knower” and “what is known” and reducing the possibility of bias. We can all see the logic in separating yourself as much as possible from your study so as not to bias it, even if we know we cannot do so perfectly.
However, the criticism often made of positivism with regard to human and social sciences (e.g. education, psychology, sociology) is that positivism is scientistic; which is to say that it overlooks differences between the objects in the natural world (tables, atoms, cells, etc.) and the subjects in the social work (self-aware people living in a complex socio-historical context). In pursuit of the generalizable truth of “hard” science, it fails to adequately explain the many aspects of human experience don’t conform to this way of collecting data. Furthermore, by viewing science as an idealized pursuit of pure knowledge, positivists may ignore the many ways in which power structures our access to scientific knowledge, the tools to create it, and the capital to participate in the scientific community.
Kivunja & Kuyini (2017)[17] describe the essential features of positivism as:
- A belief that theory is universal and law-like generalizations can be made across contexts
- The assumption that context is not important
- The belief that truth or knowledge is ‘out there to be discovered’ by research
- The belief that cause and effect are distinguishable and analytically separable
- The belief that results of inquiry can be quantified
- The belief that theory can be used to predict and to control outcomes
- The belief that research should follow the scientific method of investigation
- Rests on formulation and testing of hypotheses
- Employs empirical or analytical approaches
- Pursues an objective search for facts
- Believes in ability to observe knowledge
- The researcher’s ultimate aim is to establish a comprehensive universal theory, to account for human and social behavior
- Application of the scientific method
Many quantitative researchers now identify as postpositivist. Postpositivism retains the idea that truth should be considered objective, but asserts that our experiences of such truths are necessarily imperfect because they are ameliorated by our values and experiences. Understanding how postpositivism has updated itself in light of the developments in other research paradigms is instructive for developing your own paradigmatic framework. Epistemologically, postpositivists operate on the assumption that human knowledge is based not on the assessments from an objective individual, but rather upon human conjectures. As human knowledge is thus unavoidably conjectural and uncertain, though assertions about what is true and why it is true can be modified or withdrawn in the light of further investigation. However, postpositivism is not a form of relativism, and generally retains the idea of objective truth.
These epistemological assumptions are based on ontological assumptions that an objective reality exists, but contra positivists, they believe reality can be known only imperfectly and probabilistically. While positivists believe that research is or can be value-free or value-neutral, postpositivists take the position that bias is undesired but inevitable, and therefore the investigator must work to detect and try to correct it. Postpositivists work to understand how their axiology (i.e., values and beliefs) may have influenced their research, including through their choice of measures, populations, questions, and definitions, as well as through their interpretation and analysis of their work. Methodologically, they use mixed methods and both quantitative and qualitative methods, accepting the problematic nature of “objective” truths and seeking to find ways to come to a better, yet ultimately imperfect understanding of what is true. A popular form of postpositivism is critical realism, which lies between positivism and interpretivism.
Is positivism right for your project?
Positivism is concerned with understanding what is true for everybody. Social workers whose working question fits best with the positivist paradigm will want to produce data that are generalizable and can speak to larger populations. For this reason, positivistic researchers favor quantitative methods—probability sampling, experimental or survey design, and multiple, and standardized instruments to measure key concepts.
A positivist orientation to research is appropriate when your research question asks for generalizable truths. For example, your working question may look something like: does my agency’s housing intervention lead to fewer periods of homelessness for our clients? It is necessary to study such a relationship quantitatively and objectively. When social workers speak about social problems impacting societies and individuals, they reference positivist research, including experiments and surveys of the general populations. Positivist research is exceptionally good at producing cause-and-effect explanations that apply across many different situations and groups of people. There are many good reasons why positivism is the dominant research paradigm in the social sciences.
Critiques of positivism stem from two major issues. First and foremost, positivism may not fit the messy, contradictory, and circular world of human relationships. A positivistic approach does not allow the researcher to understand another person’s subjective mental state in detail. This is because the positivist orientation focuses on quantifiable, generalizable data—and therefore encompasses only a small fraction of what may be true in any given situation. This critique is emblematic of the interpretivist paradigm, which we will describe when we conceptualize qualitative research methods.
Also in qualitative methods, we will describe the critical paradigm, which critiques the positivist paradigm (and the interpretivist paradigm) for focusing too little on social change, values, and oppression. Positivists assume they know what is true, but they often do not incorporate the knowledge and experiences of oppressed people, even when those community members are directly impacted by the research. Positivism has been critiqued as ethnocentrist, patriarchal, and classist (Kincheloe & Tobin, 2009).[18] This leads them to do research on, rather than with populations by excluding them from the conceptualization, design, and impact of a project, a topic we discussed in section 2.4. It also leads them to ignore the historical and cultural context that is important to understanding the social world. The result can be a one-dimensional and reductionist view of reality.
Exercises
- From your literature search, identify an empirical article that uses quantitative methods to answer a research question similar to your working question or about your research topic.
- Review the assumptions of the positivist research paradigm.
- Discuss in a few sentences how the author’s conclusions are based on some of these paradigmatic assumptions. How might a researcher operating from a different paradigm (e.g., interpretivism, critical) critique these assumptions as well as the conclusions of this study
- Uttl, B., White, C. A., & Gonzalez, D. W. (2017). Meta-analysis of faculty's teaching effectiveness: Student evaluation of teaching ratings and student learning are not related. Studies in Educational Evaluation, 54, 22-42. ↵
- Benton, S. L., & Cashin, W. E. (2014). Student ratings of instruction in college and university courses. In Higher education: Handbook of theory and research (pp. 279-326). Springer, Dordrecht. ↵
- Clayson, D. E. (2018). Student evaluation of teaching and matters of reliability. Assessment & Evaluation in Higher Education, 43(4), 666-681. ↵
- Clayson, D. E. (2018). Student evaluation of teaching and matters of reliability. Assessment & Evaluation in Higher Education, 43(4), 666-681. ↵
- Boring, A., Ottoboni, K., & Stark, P. (2016). Student evaluations of teaching (mostly) do not measure teaching effectiveness. ScienceOpen Research. ↵
- Uttl, B., & Smibert, D. (2017). Student evaluations of teaching: teaching quantitative courses can be hazardous to one’s career. Peer Journal, 5, e3299. ↵
- Heffernan, T. (2021). Sexism, racism, prejudice, and bias: a literature review and synthesis of research surrounding student evaluations of courses and teaching. Assessment & Evaluation in Higher Education, 1-11. ↵
- Koerth, M. & Thomson-DeVeaux, A. (2020, August 3). Many Americans are convinced crime is rising in the U.S. They're wrong. FiveThirtyEight. Retrieved from: https://fivethirtyeight.com/features/many-americans-are-convinced-crime-is-rising-in-the-u-s-theyre-wrong ↵
- Burrell, G. & Morgan, G. (1979). Sociological paradigms and organizational analysis. Routledge. ↵
- Here are links to two HBSE open textbooks, if you are unfamiliar with social work theories. https://uark.pressbooks.pub/hbse1/ and https://uark.pressbooks.pub/humanbehaviorandthesocialenvironment2/ ↵
- Lin, C. T. (2016). A critique of epistemic subjectivity. Philosophia, 44(3), 915-920. ↵
- Wills, J. W. (2007). World views, paradigms and the practice of social science research. Thousand Oaks, CA: Sage. ↵
- Cohn, N. & Quealy, K. (2020, June 10). How public opinion has moved on Black Lives Matter. The New York Times. Retrieved from: https://www.nytimes.com/interactive/2020/06/10/upshot/black-lives-matter-attitudes.html ↵
- Bautista, M., Bertrand, M., Morrell, E., Scorza, D. A., & Matthews, C. (2013). Participatory action research and city youth: Methodological insights from the Council of Youth Research. Teachers College Record, 115(10), 1-23. ↵
- Constance-Huggins, M., Davis, A., & Yang, J. (2020). Race Still Matters: The Relationship Between Racial and Poverty Attitudes Among Social Work Students. Advances in Social Work, 20(1), 132-151. ↵
- Burrell, G. & Morgan, G. (1979). Sociological paradigms and organizational analysis. Routledge. Guba, E. (ed.) (1990). The paradigm dialog. SAGE. ↵
- Kivuna, C. & Kuyini, A. B. (2017). Understanding and applying research paradigms in educational contexts. International Journal of Higher Education, 6(5), 26-41. https://eric.ed.gov/?id=EJ1154775 ↵
- Kincheloe, J. L. & Tobin, K. (2009). The much exaggerated death of positivism. Cultural studies of science education, 4, 513-528. ↵
a statistical model that links unobservable theoretical constructs, operationalized as latent variables, and observable properties—i.e., data about the world
The value in the middle when all our values are placed in numerical order. Also called the 50th percentile.
describes an individual’s world view and the position they adopt about a research task and its social and political context
a single truth, observed without bias, that is universally applicable
one truth among many, bound within a social and cultural context
assumptions about what is real and true
assumptions about how we come to know what is real and true
quantitative methods examine numerical data to precisely describe and predict elements of the social world
qualitative methods interpret language and behavior to understand the world from the perspectives of other people
assumptions about the role of values in research
a set of common philosophical (ontological, epistemological, and axiological) assumptions that inform research
a paradigm guided by the principles of objectivity, knowability, and deductive logic